Recently, Funraise CEO and Co-founder, Justin Wheeler, sat down to talk A.I. with an expert in the field, Wes Moon. As an expert in machine learning, Wes knows that without deep analysis, artificial intelligence can be a self-fulfilling prophecy, meaning that we all see what we want to see, whether it's a muffin or a pug. Wes explained what A.I. is and is not, broke down the value of structured data patterns, and drew a starting line for beginning machine learning.
We're so stoked that Wes shared some of that machine learning learning with us that now we're going to share with you. Read on for a summary of Wes and Justin's conversation, and get your machine learning on!
Justin Wheeler: Tell us your story. Where are you from and why do you care about A.I.?
Wes Moon: I guess I would go back almost 20 years. I got into fundraising largely by accident. My friend worked at the University of Toronto and said, "Hey, do you want a job? They're hiring people." And basically they were looking for young people.
I knew something about computers. They had a CRM. They were building an SAP application and building a fundraising module attached to it. And when I got hired, you could actually look at a donor in the CRM, but you couldn't actually see any giving information or contact information. So it was pretty early days in building that CRM and I spent 10 years at the U of T.
And for the listeners in the South, this is similar to Michigan State in size. And when I started, we were raising somewhere in the vicinity of $40 or $50 million. Seven, eight years later, we had achieved $110 million. And they're now raising a couple hundred million dollars every year. Large public research institution.
And luckily enough, there was my introduction, I guess, to A.I.; my boss had dropped off an article in the Chronicle of Philanthropy, which was how banks were using predictive models to find people for wealth management. And she said, "Can you do this?" "I don't know, I'll give it a shot." Did a little bit of research, build the model, learned that if someone had a middle name [noted in the CRM], they are more likely to make a major gift.
Of course, all our major gift donors had a middle name because the researchers put it into the CRM. So you didn't really need anything too smart to get that done. I then spent really like the next 10 years working as a consultant at a couple of other fundraising shops and decided to make the move over to Blackbaud to learn more about technology.
It was there where one of our jobs was to help build out some technology partnerships so that more things could connect to their CRM. Drank the Kool-Aid and then started my own business. And really what we wanted to do was to be able to provide good predictions to all charities.
So if you think of what we did at U of T or some of the larger national hospital organizations, they have a lot of processes. They have a lot of people who do a lot of math in the background to help identify good prospects and move them through a pipeline. And we wanted these predictions to be able to do that for fundraisers at scale. So, you don't necessarily need to be raising $50 million dollars to be able to do that anymore.
Justin Wheeler: When looking at predictions or predictive model, what are some of the key data points that help you predict whether or not a donor is ready to give?
Wes Moon: Humans are surprisingly predictable, actually, Justin. I'll say that, unsurprisingly, past giving is the best predictor for future giving. And that's not always the case with every individual. But en masse, if you made a previous gift, you're more likely to make another gift.
And then you start to get into a lot of different ways to look at this. Now, the way A.I. works, is that it does pattern recognition on an individual basis. And the data that we look at is structured versus unstructured. So, unstructured would be a picture. It would be consuming our conversation and changing that into text. Structured is looking at data patterns within a database, for instance, and identifying pattern recognition and extension.
So when we look at what we're doing, we're doing that structured data approach and we're using it to identify that pattern, which almost always starts with the previous gift. But then, what is the next most important variable for any one of those individuals? There are some cases for certain where the past gift isn't the most important variable. Perhaps the most important variable is this person's net worth because that's off the charts. Because they made a small gift, we may be making a prediction of a larger gift next time.
Justin Wheeler: What is A.I.; define the term for us so we make sure we all are on the same baseline.
Wes Moon: I'll start first with what A.I. isn't. A.I. stands for artificial intelligence, and M.L., machine learning, is not generalized intelligence. We're not building a robot. We're building a machine that will consume some data and produce a couple of outputs. It learns over time and it learns from your data.
You take a set of data and you look at positive outcomes and you look at negative outcomes. I ask Justin for a gift; Justin made a gift. That's a positive outcome. And then we look at Justin's friend; Justin's friend didn't make a gift—That's a negative outcome.
So you're always trying to pull the curve up and down for every individual. If you think of it in math, it's almost like a graph. Where is this graph going? What is the equation for any individual as it pertains to the next gift? And when we think of A.I. today, it's very much about answering a very specific question.
The U of T, actually the birthplace of A.I. or modern A.I., had a contest. Is it a pug? Is it a muffin? That's a very specific question. And for about ten years, a computer couldn't beat a human from telling an image of a pug versus an image of a muffin. Surprisingly, if you Google it, they look very similar. Now, what allowed that to happen is the training. Some guy sat in his basement, I assume there's a basement, maybe a garage and clicked through and taught a computer just by saying, choose one. Which one's a pug? Which one's a muffin? And over time, it built up a repository of information to be able to tell the difference.
It's that learning aspect oh A.I. that's very important here.
Justin Wheeler: So with the predictive models that you've built at Wisely, I would presume that the more data that you're getting, the smarter they're becoming. Maybe accurate is not the right word, but just the more robust they're becoming. Would that be a correct assumption?
Wes Moon: Yeah, totally. So the longer we work with the client, the more accurate the data becomes. But also, the more clients we work with, the more accurate the data becomes. Sure. We're not commingling data, per se, but the learning is centralized into one brain.
Justin Wheeler: Where does a fundraiser get started? Where would you recommend someone get started? Or if I were to onboard with Wisely, what does that process look like and how do we start using A.I. in our fundraising strategy?
Wes Moon: It's probably easier to talk about what we would do first and then there are some other options to consider. We need to connect to your CRM. So that's typically the heavy lifting job that we would need to do. Depending on your CRM.
We've already built up the integration and we take all your data and then we run it into our machine. And our machine thinks for about 24 hours, depending on how much data you have. So some of our larger clients, it takes 24 hours, some of our smaller clients, it can actually just take minutes if they only have 5,000 donors or 10,000 a year.
And we then produce an output every day because data changes, time changes. And the concept is next gift amount. Next gift date. So when you start to think of what could you do if you knew every donor's next gift amount or next gift date, there is a lot. We are very focused on using these two predictions today.
But where we want to go into the future, and this is entirely doable, is creating a customized donor plan for every single person you've ever met. Is this person an event donor? Is this person a planned gift donor, or will they make it in the estate? Do you need to assign a stewardship officer to this person making estimated lifetime value? So all those things are possible to predict using A.I. and that structured data with the data that almost every charity has if they're using a CRM.
Justin Wheeler: Yeah. So on those two concepts, next gift amount and next gift date. Is that meant to be a mark, or like an indicator for the fundraising team?
Wes Moon: Yeah. Generally speaking, we are using it for people making larger gifts.
Depending on our client, that might be $500. That might be at $1,000. That might be at $5,000 or $10,000, depending on their sophistication in their major gift program.
What we then do is advocate for a process. So I told you that story about U of T where I built my model. I got better at building models. I no longer build models. I have mathematicians for that now. What we always struggled with was to figure out who should we actually spend time with, what relationship should we build? Because as fundraisers, time is always of the essence. We don't have enough.
And we always struggled to put that into practice. So the advancement services team or the operations team would come up with some good recommendations and that would go to gift officers and gift officers would go back to the way they always did their job, which was talking to the people that they know, doing a little bit of outreach to the people that they don't know. And it felt more secure for them to be able to hit their goal that way, because it's the way they had always done it.
So we wanted to build a product into this. So behind the scenes, we have our prediction engine that's making these pretty cool predictions. So the first tool that we've built is a prospecting tool. So most organizations have someone whose job it is to try to find some of those donors who look like they could become major gift donors. So we mine the database for the person.
We provide a tool that allows that operations person or sometimes that fundraiser to assign a donor to their portfolio. And then we built a prioritization tool to say, "When should I reach out to this person? How much should I be thinking about in terms of qualifying this donor for? What does my opportunity look like?"
And the very cool thing for some of these fundraisers and operators, you have a portfolio. A typical portfolio in the fundraising world is about one hundred people, give or take. You can tell if your portfolio supports your goal with a reasonable degree of accuracy on the first day of that year. We're picking a gift amount and a gift date.
And with those two pieces of information, it becomes a very powerful tool to plan your year, to plan your quarter, to plan your month, to plan your day. And that's about prioritizing who you spend time with.
Justin Wheeler: It sounds like it also brings a ton of efficiency into just the whole cultivation process of major donors in particular. And if you have these predictions, you have these dates, then you know exactly who and when to be talking with at the right time. And that sounds like that's exactly what this helps a fundraiser do.
Wes Moon: Totally. And that's what's very interesting, and this is a byproduct of what we build, is that the best time to thank a donor is right after they make their last gift. But most charities don't have the resources to thank every single donor after every single gift with a live human being. But if you see someone who makes a small gift, but their next gift appears to be quite large, throw them right into a portfolio and you get to start with stewardship right after they made their last gift. It's a nice touch.
Justin Wheeler: Yeah. Is this all part of the Wisely product? Like, you have a portfolio tool that will bring the donors into the portfolio and show this information? Or is this through the integration with the CRM?
Wes Moon: You can do it either way because we have deep integration. You can use your CRM to do it or you can use our product to do it. Many CRMs have different capabilities, so we needed to build some core functions to make sure that people can use the data.
Justin Wheeler: Got it. So we've talked about, you know, a couple of the positive concepts. You know, when thinking about A.I. as you've built out the product and as you've gotten deeper into the A.I., predictive modeling for fundraising, are there other concepts that just don't work well with A.I. or things that fundraisers should stay away from when they think about A.I. and fundraising?
Wes Moon: I guess I would say there's a general warning. And that general warning would be true of almost all A.I. And it's it involves bias.
So bias, as it pertains to A.I., is generally based on the process that exists at an organization today. So when you're looking at your data and you're doing pattern extension, the data reflects the process that exists at an organization. So if I'm a school, let's say, and I'm only talking to Engineers, the A.I. is going to propagate that. It's going to say, well, you need to talk to all the engineers all the time because they're the best. They make the best guess of the best donors. You need to have more events for them because of the people who attend. And that can become a self-fulfilling prophecy where if there's a process that goes into it and you're not careful about what that process is and what desired outcome you have, you can get into a death spiral.
So we have this concept where we wanted to be able to recommend the next action in a very simple way. And what we found is that the next best action was an email always because the email was the most common. And then because it was the most common and recommended, there's more e-mail. So we had to actually not use that and go with the concept of it has to be a real piece of product. You can't do it in a light way. You can't, otherwise, bias will get you.
Justin Wheeler: Got it. That makes sense and you have to then start to unlearn the biases. So is there a sort of threshold where it doesn't make sense for a nonprofit to use machine learning because they don't have enough data? Or can they leverage the learnings of other people's data and apply it to their own sort of donor file?
Wes Moon: If you don't have a couple years of 10,000 donors coming in, it would be very difficult for an organization, even if they had the dollar resources, to actually be able to build their own A.I. product.
You just don't have enough data for it to be statistically significant. If you're going with someone who's productized A.I., and there's a few of us out there who are doing it, that starts to become a little bit easier because you've got a model that has been trained on a similar dataset and you just throw your data into it. So you may not have enough data to actually train, but there's probably enough for it to be directionally accurate. And we're trying to be able to support charities that have 5,000 gifts a year 2,000 gifs a year. We want to be able to support you. So we've designed our A.I. model to be federated so that we should be able to do that.
Justin Wheeler: When organizations bring their data to you, do they have to purchase more data to kind of get more detail? So if an organization is not doing any sort of wealth scanning of their donors today, when they come to you, is that something that you do to enhance the data, or do they have to purchase the data to make the machine learning more effective?
Wes Moon: In both Canada and the United States, there is an absolute treasure of free data available, and the beautiful piece of it is that it's not personally identifiable. So everything that we do is not personally identifiable when we append any of this data. We think that's the right privacy approach to take. And with that supplemental free data that's available, that allows us to get to about 90%, give or take, observed accuracy.
So if you purchased some supplemental data, which we've tested with, it does improve the accuracy a little bit, in particular on the outliers.
Justin Wheeler: At Funraise what we're doing some machine learning, not yet on predicting the next donation date or amounts; more on the fraudulent side. We recently released a model that's helping detect fraudulent transactions across the Funrise ecosystem.
And it's been fascinating to see the effectiveness of it and understanding what are the characteristics of a fraudulent transaction. But I think there's a lot of opportunity for us to partner on the fundraising side because what you're talking about would benefit a lot of our customers. And there's a lot of data that would bring a lot of value to the Funraise customer base in terms of the concept that you guys are focused on as it relates to outcomes.
Wes Moon: It's very effective. I mentioned humans are very predictable. We have a partner who we're hoping to do a pilot project with on personality. So with just a couple hundred words, you can actually predict someone's Ocean score. I'm not sure if you're familiar with Ocean. It's a personality matrix. A little bit like Myers Briggs. But each one is a linear scale. So openness, conscientiousness, extroversion, agreeableness, and neuroticism.
Justin Wheeler: That actually kind of leads to my next question: What are some other areas that you guys are interested in building machine learning around as it relates to fundraising and nonprofits in particular? Is there anything else that you guys are noodling on or thinking about that would bring a nice uplift to organizations trying to scale and raise more?
Wes Moon: When we think of our grand vision, if you will, of being able to provide a donor plan for every single constituent that you've worked with...
Looking at lifetime value. So what we then need to do is to we need to be able to work with the event folks. We need to be able to work with the direct marketers and the digital marketers to say, "These are the types of engagements that this audience needs." And being able to cohort data for the clients, because that's a lot of hard work. And we're not... we don't understand the communication of the charity and how they actually speak to their donors. But what we can do is identify and group people so that they can focus on their mission, and we do the math in the background.
When we think about the direction that we're going, that's very much a focus. We've built a couple of light models for some of our clients who want to do some monthly donor conversion, for instance, on gift file. And we're actually doing some work with some of our clients right now about looking at event participants and event sponsors in that peer-to-peer space and seeing if there's some good ways to actually monetize that while they push off an event for a year.
Justin Wheeler: Interesting. Very interesting. Where do you see your company going on the front end of digital fundraising, whether it's like within peer-to-peer or website giving, do you see a need for more enhanced machine learning on that side, like, changing button colors, changing images, changing copy, depending on what you know about the individual visiting your sites? Anything there that you think could be interesting to help organizations see higher conversions on web traffic, for instance?
Wes Moon: The people who are going to solve that are the people who are very focused on digital marketing. So when we think of segmentation and plans and what are we doing with this donor, that's where we need to live.
To help predict what is the best case, there's a company that I do some work with in Toronto. They're a startup and they're doing digital storytelling as a part of their A.I. projects. So it's actually taking the messages that are going out and looking at them and saying this is how to optimize it.
The A.I. space is so specialized. And the question that you want to use your model to answer is so specific, that you wouldn't want to be so divergent in your approach.
Justin Wheeler: I'd love to hear your thoughts around what is a fundraising team missing out on by not adopting A.I. Into their practice. I mean, it's very straightforward. Like what am I missing out on by not, you know, leveraging A.I. for our fundraising activity?
Wes Moon: If you're today not using A.I., you're going to fall behind. That's the very simple truth. And maybe the best way to describe it. Justin, I don't know if you're old enough to remember not having even MapQuest, for instance.
Justin Wheeler: I remember printing MapQuest, you know, or printing directions. You got the directions and you printed them and you took them in the car with you because you couldn't have them on your phone.
Wes Moon: Totally. So before MapQuest, you used to go to AAA, the Auto Club, and they would do the map for you and print it out. And then MapQuest came out and it blew everyone's mind. I used it to drive to San Diego, from Toronto. MapQuest. Printed out. It was amazing. And then now, we would never think of doing that. Our phone is actually telling us turn by turn, where to go. Avoiding traffic.
And not only that, it's telling us when to leave. And when we think about using A.I. and fundraising, it's the same thing. We could get to our destination. But what we need to do is elevate ourselves for higher-level activity. If we're using this car analogy, how do I get there safely? How do I know I'm getting there on time? Do I have everything that I need? And then it also removes a giant burden from you on the administration.
So when you think of how that can have an impact on an organization, you start to really say it's almost an essential for you. Banks are going crazy with this. Like large organizations are and have been investing in this for quite some time. It's half of Amazon's business. It's half of Google's business. It would be naive to think that we shouldn't be looking at this and finding ways to short cut our way through our businesses.
Justin Wheeler: Yeah, I think that the analogy, the car analogy is so right on. So when an organization or when an individual is donating, we're pulling in demographic information, wealth information that's not being obviously entered on the form. And I've noticed that some nonprofits have a really hard time accepting that as good practice. They feel like it's unethical to get this information. And I imagine there may be even some organizations that have the same similar feeling to A.I. Do you run into at all within your clients or nonprofits that you've spoken to? Just not thinking about A.I. as more of an evil sort of part of technology versus something that's positive that can actually drive much better results for the mission.
Wes Moon: I would say when we started our business a couple of years ago, two and a half years ago at this point, we were very focused on bringing A.I. to the charitable sector. It was generally received as, "You guys are nuts." There's a general concern that the data that a charity has isn't good enough for it, which is entirely not true. I can tell you that I've looked at plenty of data sets for even Fortune 500 companies that don't compare to most charities. Charities are mandated by the government for good record keeping. So believe me when I say their data is actually pretty good, despite the fact that the grass looks greener elsewhere.
And I think those two things, coupled with a very tricky situation that I think most charities and fundraisers find themselves in, is that they care very deeply about the good work that they're providing. Donors specifically want all their donation gift amount to go towards the good works and they have to decide, how do I reconcile these two things and spend money on something that makes my job easier?
Maybe my job isn't supposed to be easier. It's hard for me to actually know. And the sort of look that cost-per-dollar raised, coupled with data, coupled with, "Maybe this will put me out of a job," which is not true at all. In fact, hopefully, what we find is that we're able to actually help charities understand how many gift officers and prospect researchers their database will support as opposed to retracting.
Justin Wheeler: Totally. Well, thank you Wes so much for sharing. I think what I really appreciate about the content today is you really broke down and made A.I. less scary for nonprofits. And just talking about the simple concepts of, "What are the outcomes we're trying to achieve?" We're trying to help you understand when and how much to ask from your donor base that exists today. So thank you so much for coming on the podcast to share with us your expertise. How can people get in touch with you if they'd like to learn more?
Wes Moon: We're at fundraisewisely.com or wisely.ai. Go to our website. You can reach out to us there.
Justin Wheeler: Awesome, Wes, thank you again for joining.






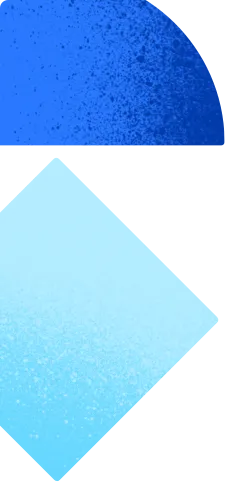

